The world community faces increasing flood risks as the pattern of flooding on all continents is changing and becoming more frequent, intense and unpredictable. Floods are the most devastating type of natural disaster affecting human lives around the world [1]. The impact corresponding to urban floods is significant due to factors such as high population density, large impermeable areas, and obstruction of drainage systems. The impact caused by flooding in urban areas can be physical, economic, social and environmental [2]. One of the most important indicators of the consequences of such hazards is measured by the high level of economic losses that are usually expressed as direct economic damages [3].
The word risk is used in several contexts. It is related, for example, to the environment, economy, and engineering discussions, among others. The attempt to give a single definition to risk, applicable to all areas, is not advisable in view of the difficulty in addressing different and specific aspects of each one. Therefore, it is usual and necessary to adopt a particular interpretation for risk analysis, consistent with the topic addressed [4].
The risk concept used by United Nations International Strategy for Disaster Reduction (UNISDR) [5] defines risk as the probability of harmful consequences or expected losses (deaths, injuries, interrupted economic activities or environmental damages) resulting from interactions between natural or induced risks vulnerable conditions of a socioeconomic system. Conventionally and in a simple way, the risk can be expressed by the relation: Risk = Hazard × Vulnerability × Damage.
Veyret [6] conceptualizes risk as perceiving a possible danger, more or less predictable by a social group or by an individual who has been exposed to it and can suffer from it. It can be emphasized that the hazardous event alone does not define the risk. It is necessary that the individual or social group has to be exposed and vulnerable to the hazard. Risk is inscribed within a social, economic and cultural context, therefore, it is not possible to examine risk representations without considering management practices.
It is important to highlight the concepts of susceptibility and social vulnerability, which are necessary for the discussion and analysis of flood risk. According to Alliance Development Network and United Nations University, Institute for Environment and Human Security (UNU-EHS) [7] susceptibility refers to conditions of exposed communities or exposed elements (infrastructure, ecosystems, etc.) that become more likely to experience damage and be adversely affected by a natural disaster.
For Intergovernmental Panel on Climate Change (IPCC) [8] vulnerability is the degree to which a system is susceptible and unable to cope with adverse effects of climate change (which is the particularly concern for IPCC), including climate variability and extremes. The social vulnerability can be expressed through socioeconomic and demographic parameters. The vulnerability is related to the social disadvantages that are reflections and products of poverty, which, in turn, is understood as a social condition that restrictively affects individuals, social groups, and places. In this sense, vulnerability corresponds both to limited access to knowledge and to the low capacity and inability to manage the resources and opportunities available to society for the development of its members. It is also reflected in the inability to recover from damages. This conceptual vision allows anticipating risks and potentiating eventual adaptations.
In the face of frequent flooding, it is necessary to improve the retention and infiltration of rainwater in urban river watersheds [9]. However, the traditional solution for urban stormwater problems in most countries refers to structural interventions involving storm drains, canals, and dams aiming to avoid local flooding. An usual consequence of this approach is that, by increasing the conveyance and flow velocity, it can change the river dynamics and transfer problems downstream [10]. A new approach is needed that includes both structural and non-structural measures, recovering natural responses, and maximizing multifunctional opportunities for land use [11].
However, cities located on riverbanks, in naturally fragile areas, subjected to flood risks (and residual flood risks) may require a management approach also based on resilience to floods and not only on resistance. Resisting floods through dams, channels and storm drains neglects inherent uncertainties stemming from human-nature interactions and does not address the extreme events that are expected to increase with climate change and therefore is not a reliable approach to face long-term flood safety demands [12].
Urban floods cannot be managed separately in the city scale and the responses to the possible impacts of the flood are complex because of political and socioeconomic issues linked to environmental changes. To understand the characteristics of the effects of urban floods, a space-time structure must be developed to clarify the causes of the vulnerability of cities [13]. According to Bobrov [14], urban planning is the design and regulation of space use which focus on the physical form, economic functions and social impacts of the urban environment and on the location of different activities within it.
Tapia et al. [15] developed an indicator-for vulnerability assessment in European countries, based on potential hazard-receptor combinations (impact chains) (i.e., heatwaves, drought, and flooding). The vulnerability assessment can be used to allocate the international cooperation resources targeted to adaptation plans.
It is widely recognized that flood plains have always been attractive settlement areas. The time and space pattern of flood flows, and the exposure and vulnerability socioeconomic system define the risk. Exposure is dependent on the spatial extent of the hazard that threatens the population. Its magnitude and frequency is related to with the vulnerability [16]. The importance of integrating water management in urban planning to promote city resilience to flooding is emphasized in this context. Rapid and preliminary assessment of possible economic losses caused by outflows in an urban basin is very important for adopting disaster mitigation measures [17]. Disaster management is a process that includes activities before, during and after a risk event that aims to reduce disasters impacts and to recover from their losses [18].
The risk is very often evaluated subjectively, taking into account only a few factors that contribute to the problem and leaving aside other factors that affect its criticality. According to Ranzi and Vu [19], the quantification and assessment of flood damage is an important factor to be considered in the decision process to define specific measures for flood risk management. Risk assessment through the formulation of an index allows the introduction of a quantitative measure, although not precise, that is able to join different subjective matters and map the importance of a given risk.
The formulation of an index is given by the mathematical equation that expresses the relations between the indicators that compose it intending to represent what one wishes to measure. Its construction, in turn, aggregates information associated with indicators of different meanings and natures, translating them into a single representative value of reality, thus allowing comparisons in time and space [20]. On the other side, choosing the indicators adequately is crucial to the effective representativeness of the index.
For Kim [21], indices are necessary for any information collection, and the function of an index is to provide users with systematic and effective shortcuts to the information they need. Thus, indices can perform diverse functions, such as evaluating existing conditions, comparing places, situations or alternatives, providing advance notice of any effect or impact of an action and predicting future conditions and trends.
An index allows aggregating information associated with indicators of different constitutions, translating them into a single (and simple) value representative of a real complex situation. According to Alegre et al. [22], the process of creating these indicators and/or indices includes obtaining elementary data, which are filtered and transformed into variables that are combined to form indicators (and indices). Its ultimate goal is to provide relevant information to be used in the decision-making process especially in early planning or comparing alternative choices. Multi-criteria methodologies can play a very important role in the formulation of indices.
Geographic Information System (GIS) tools and mathematical modeling can also be used in this type of study. A GIS may be used to retrieve and exploit the spatial properties of the visual structure inherent to space [23]. GIS is an emerging tool for planning and application of projects to territory [24]. Thus, GIS provides better information for decision-making situations [25].
Mathematical modeling allows the simulation of real systems in order to predict their behavior. In the absence of sufficient historical observations about the floods, advances in remote sensing and computational power have created more attractive mathematical models, where spatial predictions of potential damage from future flood episodes are calculated [26]. Currently, mathematical modeling methods are effective tools for studying non-stationary processes found in the natural environment [27]. When combined with multi-criteria approaches, the mathematical models, which are usually more sophisticated, more expensive and more data and time consuming, may be used in a preliminary phase intending to calibrate and or validate the proposed index.
In this context, the main objective of this work is the development of a multicriteria methodology for flood risk analysis, to aid urban planning and to support flood risk management policies, in order to avoid the occupation and densification of areas susceptible to flooding. The Inundation Risk Index (IRI) seeks to aggregate simple indicators, combining physical characteristics of the river basin, related to the natural propensity to flooding, and the social features of the affected population.
The IRI was developed to identify, in a relatively simple and fast way, the flood risk areas of a basin. An evaluation of its effectiveness was made using the case of the Bacanga River basin, in the municipality of São Luís/Maranhão State, in the northeast region of Brazil. The IRI is composed by two sub-indices, one responding by the physical susceptibility to flooding, the other representing the social system vulnerabilities. The hydrodynamic model called MODCEL, developed by Miguez [28], was used to validate the susceptibility sub-index, comparing flooded areas modeled with those mapped by the sub-index. The methodological steps are described in the sequence following below.
Geoprossessing tools were used to manipulate and agggreate information in the watershed space. Figure 1 briefly shows the geoprocessing steps developed as a preliminary activity to operate the IRI.
Procedure flowchart

The formulation of an index is given by the mathematical equation that translates the relations between the indicators that compose it [29]. In the case of flood risk areas, the formulation should reflect the interaction between two sub-indices: physical susceptibility to flooding and social vulnerability.
Each sub-index consists of several indicators, each of which brings a specific information. According to Jonkman et al. [30], numerous definitions of damage exist. The categorization of damage into direct (related to physical contact of water) and indirect, or into tangible (quantified in monetary terms) and intangible is commonplace, but interpretations and delineations of categories differ. The choice of indicators takes into account the damages and constraints that may be caused by floods, such as fatalities, direct and indirect damage to the health and physical integrity of individuals, and physical damage to the buildings structure.
The sub-index physical susceptibility to flooding is associated with the physical characteristics of a watershed, such as altimetry, slope, pedology, and vegetation. According to Elshorbagy et al. [31], these factors are the most determinant in this type of study. According to Shutzer [32], the flood plains function storing waters, which contributes to reducing the probability of local flooding, once these areas are not occupied.
In the other hand, the social vulnerability sub-index cannot be determined so objectively, because it refers to the exposure of people and goods likely to be affected by a flood, and may assume a multiplicity of interpretations and biases [33]. In this study, the indicators age, schooling, income, population density and subnormal groupings were used as a way of approaching the vulnerability assessment of low resilience characteristics, considering a social bias.
IRI uses a mixed weighted formulation, consisting of a product of two summations. This mixed weighted formulation is similar to that proposed by Zonensein [29]. The general IRI formulation can be seen in eq. (1):
(1)
where IRI is inundation risk index, ISUP is sub-index of susceptibility and IVUL is sub-index of vulnerability.
Each sub-index consists of several indicators, each with an importance represented by its weight. Only if all the indicators that make up a sub-index are null will it also be null. Weights ranging from 0-100% (0-1) were defined for each variable in order to differentiate the degree of importance and correlation with the phenomenon. Thus, each of the sub-indices is calculated from a weighted sum of previously normalized indicators with scores varying from 1 (minimum) to 5 (maximum), as shown in Table 1.
Standardization of indicators
Note | Classes |
---|---|
1 | Very low |
2 | Low |
3 | Medium |
4 | High |
5 | Very high |
The adoption of weights for each indicator, in the final composition of the index, is an easy task, which depends solely on technical knowledge, as it also results from the perception of the risk of the evaluator and the bias that is intended to be observed importance. Therefore, it is recommended that the allocation of weights be performed by the manager or by the institution planning and using the index to support the decision flood risk management.
In this work, the applied method is the peer-to-peer Analysis Hierarchical Process (AHP) created by Saaty [34]. It is a method widely used in the context of multicriteria analysis and decision support systems, because it not only allows identifying the best option within the possible alternatives, but also helps in the determination of priorities, considering qualitative aspects and/or quantitative measures.
The determination of the standardization scales of the indicators, consider the established domain for the index. If the scales were indicated considering a small domain (e.g. sub-basin) they should be reviewed before application in larger domains. In the case of linear metric scales, the more favorable and less favorable indicators should take into account the entire field of index. This consideration also applies to the determination of limits on the scale of standardization by bands.
Thus, if the scales are fixed, general comparisons can be made (regional, national or international), however, sensitivity may be lost if the objective is to make a local decision or if it is a municipality that wishes to evaluate their situation. Adaptations in the scale make the value of the index lose the generality, but allow local observations and conclusions of greater detail and quality. In any case, the general methodology is always valid, even with scale adjustments.
The susceptibility to floods in a given area is directly related to aspects of geomorphology, pedology, land use and occupation and hydrography [35]. Table 2 shows the specific weights adopted for physical indicators considered for the Bacanga River basin application.
Indicator weights
Indicators | Weight (0-1) |
---|---|
Vegetation | 0.15 |
Pedology | 0.15 |
Altimetry | 0.20 |
Slope | 0.50 |
This weight distribution makes explicit the assumed importance of the slope indicator, with this variable being 50% of the total weight-flatter areas are susceptible to flooding, while steeper areas do not accumulate water. Therefore, rain waters tend to occupy the lower areas and to undergo temporary storage in areas with smaller slopes. The weight of 20% was attributed to low altimetry, since it is an important factor in coastal areas subjected to tide effects, as it happens to be in this case study. Note that in non-coastal areas, the weight of this indicator may be reduced or even equated to zero. Vegetation and pedology had a weight of 15%, both dividing the remaining weight values.
The final formulation for this sub-index is given in eq. (2), which reproduces the first part of eq. (1), already with the weights assigned, according to the particular interpretation of this work to the case study application:
(2)
where ISUP is physical susceptibility to flooding sub-index, SLO is slope indicator, ALT is altimetry indicator, PED is pedology indicator, and VEG is vegetation indicator.
The normalization of the indicator for Slope considers the influence of different ranges of slopes on the probability of flood occurrences. The following ranges were adopted, as shown in the Table 3.
Standardization of indicator Slope
Variable | Note | Classes of flood susceptibility |
---|---|---|
> 5.7% | 1 | Very low susceptibility |
4.3% < quota <= 5.7% | 2 | Low susceptibility |
2.9% < quota <= 4.3% | 3 | Average Susceptibility |
1% < slope <= 2.9% | 4 | High susceptibility |
< 1% | 5 | Very high susceptibility |
These values are associated with the case study, adapted from the slope classes of the Brazilian Agricultural Research Corporation (Embrapa) [36].
The normalization for the Altimetry indicator evaluates the effects of different ranges of altimetry dimensions on the possibility of flood occurrence, considering the influence of the tides in coastal areas. The altimetry was classified in the following classes (Table 4).
Standardization of indicator Altimetry
Variable | Note | Classes of flood susceptibility |
---|---|---|
> 10 m | 1 | Very low susceptibility |
7 m < quota <= 10 m | 2 | Low susceptibility |
5 m < quota <= 7 m | 3 | Average Susceptibility |
2 m < quota <= 5 m (zone of influence of the tide) | 4 | High susceptibility |
< 2 m (below the protection of the Bacanga dam) | 5 | Very high susceptibility |
For the Pedology the normalization was based on the evaluation of the porosity and permeability effects of each type of soil. Pedology classification considered is found in the Table 5.
Standardization of indicator Pedology
Variable | Note | Classes of flood susceptibility |
---|---|---|
Quartzite aerosols | 1 | Very low susceptibility |
Regolytic cells | 2 | Low susceptibility |
Gleissolos | 3 | Average Susceptibility |
Argisols red – yellow | 4 | High susceptibility |
Argisols red – yellow (laterites) | 5 | Very high susceptibility |
The normalization of Vegetation was based on the assessment of the effects of deforestation on infiltration, retention and surface runoff, with consequences on flood levels and consequent flooding areas. The classification of vegetation adopted is shown in Table 6.
Standardization of indicator Vegetation
Variable | Note | Classes of flood susceptibility |
---|---|---|
Forest | 1 | Very low susceptibility |
Shrub vegetation | 2 | Low susceptibility |
Pasture | 3 | Average Susceptibility |
Exposed ground | 4 | High susceptibility |
Mangrove and urbanized areas | 5 | Very high susceptibility |
The socioeconomic variables used to map population vulnerability in the Bacanga watershed were evaluated according to the compilation and analysis of data from the Demographic Census of 2010, conducted by the Brazilian Institute of Geography and Statistics (IBGE) [37]. Table 7 shows the weights adopted for vulnerability indicators in the Bacanga River basin.
Indicator weights
Indicators | Weight (0-1) |
---|---|
Education | 0.05 |
Age | 0.05 |
Income | 0.15 |
Subnormal clusters | 0.25 |
Demographic density | 0.50 |
The proposed weight distribution shown in Table 7 highlights the importance of the exposed population, through the demographic density variable. As a necessary element to configure risk, the exposure in the vulnerability computation assumed 50% of the total weight. The other 50% were distributed to typical elements of vulnerability: substandard occupations, with a 25% weight, which presents notorious poor infrastructure and urban fragilities. The income received a weight of 15%, as it is related to the material recovering power of the affected population. Education and age received equal weights of 5%, with these variables being related to the population’s responsiveness to floods, highlighting the most fragile groups, considering formal education as a positive value for rapid reactions and the situation of elderly and children as the most fragile population. These last two variables received a smaller weight, reflecting a fine adjustment in the vulnerability calculation.
The final formulation for this sub-index is given by eq. (3), which reproduces the second part of eq. (1), with the weights defined in this work:
(3)
where IVUL is vulnerabilty index, DEM is demographic density indicator, SUB is subnormal agglomerates indicator, INC is income indicator, AGE is age indicator, and EDU is education.
In determining the weights of the indicators, it was established as being the largest population density is important because it represents the number of people floods (giving a measure of exposure). For the remaining indicators, the characteristics that contribute the greatest damages and in an area affected by floods were considered, with emphasis on subnormal occupation.
It is important to stress that the chosen weights are only a particular interpretation proposed by the authors. Different places and different users may choose a different and valid set of weights.
Demographic density indicator used the population and the area reported by census tract, in the Bacanga basin, as computed in IBGE database. Therefore, the proposed classes were particularized for the case study, as shown in the Table 8.
The so-called Subnormal Agglomerates are areas without adequate urban infrastructure, which transform the entire neighborhoods into unsuitable places to live. The population living in this type of place is more vulnerable to flood damage. Here, a binary logic (yes or no) has been adopted (Table 9).
Standardization of indicator Demographic density
Variable | Note | Social vulnerability classes |
---|---|---|
0-300 hab./km² | 1 | Very low vulnerability |
301-600 hab./km² | 2 | Low vulnerability |
601-900 hab./km² | 3 | Medium vulnerability |
901-1,200 hab./km² | 4 | High vulnerability |
More than 1,200 hab./km² | 5 | Very high vulnerability |
Standardization of indicator Subnormal clusters
Variable | Note | Social vulnerability classes |
---|---|---|
No subnormal clusters | 1 | Very low vulnerability |
Subnormal clusters | 5 | Very high vulnerability |
The Income indicator considered the average monthly household income per capita as reference. The income variables were elaborated from the IBGE [38] using salary bands, which determine the social class of the Brazilian population. This is an easy and objective calculation criterion, based on the number of minimum reference wages (Brazilian reference unit) and divided into five income ranges (as the Table 10 shows).
Standardization of indicator household income per capita
Variable | Note | Social vulnerability classes |
---|---|---|
More than 10 minimum wages | 1 | Very low vulnerability |
5 to 10 minimum wages | 2 | Low vulnerability |
2 to 5 minimum wages | 3 | Medium vulnerability |
Up to 2 minimum wages | 4 | High vulnerability |
No income | 5 | Very high vulnerability |
Education is associated with the level of schooling of the population, which provides (or not) a greater capacity for reaction and, therefore, a better defense and self-protection capacity. The IBGE Demographic Census, which only reports the rate of literate and non-literate population, was used as reference. From this situation, a simplification of the scale was carried out, which again uses binary logic (yes or no), according to Table 11.
Standardization of indicator Education
Variable | Note | Social vulnerability classes |
---|---|---|
Literate | 1 | Very low vulnerability |
Illiterate | 5 | Very high vulnerability |
In the scope of the Brazilian Protection and Civil Defense reasoning, it is considered that the most vulnerable group of people is composed of “children, pregnant women, the elderly and people with disabilities” [39], as defined in Law 12,608 of April of 2012 [40]. This is due to the functional limitations that increase vulnerability in disaster situations, since the commitment of variables such as risk perception, alertness, attention, agility and mobility make it difficult to respond in these situations. Therefore, the indicator Age , as shown in the Table 12.
Standardization of indicator Age
Variable | Note | Social vulnerability classes |
---|---|---|
More than 10 minimum wages | 1 | Very low vulnerability |
2 to 5 minimum wages | 3 | Medium vulnerability |
No income | 5 | Very high vulnerability |
According to the formulation and the chosen indicators, the IRI is defined by the structure presented in eq. (4), considering general weights:
(4)
The hydrodynamic modeling was developed with the purpose of validating the proposed sub-index of physical susceptibility to flooding. A flow cell model was used, MODCEL [41], which assumes that a basin can be subdivided into a set of homogeneous compartments, called cells, which represent urban landscapes in an arrangement such that it reproduces the flow patterns, inside and outside the drainage network [42].
The modeling of the Bacanga river basin led to 196 cells, representing the main channels, a reservoir, the natural plains and the urban areas. The connections between cells are made by hydraulic laws representing discharges in channels, over spillways and the surface runoff.
The rainfall-runoff transformation is also done by MODCEL, using the Rational Method to separate the effective rainfall in each cell. The rain gauge of the National Meteorological Institute (INMET) (code 82280), located near the Bacanga river basin, at Marechal Cunha Machado airport was used as reference. The real rainfall measured on April 11, 2009, was used in the calibration process.
The occupation of São Luis privileged, in a first moment, the flat lands not subjected to the floods. With the rapid urban growth, many slopes and fluvial channels were modified by man in a disorderly way, disregarding the fluvial geomorphology and its natural processes, leading to the occupation of risk areas.
Bacanga River basin presents 63.08% of its watershed in flat relief area, with altitudes of up to 3 meters, in the fluvial-marine plains, and from 4 to 7 meters, in the fluvial plains. The absence of vegetation cover associated with certain characteristics of its soils, slope and altimetry, lead to great flow velocities, little amount of water stored in the soil and usually result in expressive floods. Figure 2 shows the map of physical susceptibility to flooding for the Bacanga watershed, spatially mapped by census tracts.
According to Coelho [43], relatively flat regions or low slopes present natural drainage difficulties.
The sub-index representing physical susceptibility to flooding may also be used separately for pre-identifying the sites with the highest degrees of flood susceptibility, with the main objective of assisting urban planning. This is not a specific or rigorous flood mapping, since the process is not deterministically modeled. Therefore, it was not intended to precisely detail the phenomenon – this is not the role of the indexes. The goal is to provide a simple tool that can quickly and consistently indicate which areas require more attention in urban planning and which require more detailed studies to house urban sprawl.
According to data from the Demographic Census 2010, conducted by the IBGE, 232 census tracts are considered subnormal clusters in São Luís, 52.58% of which are located in the Bacanga basin. In the IBGE indicator on illiteracy, Maranhão was the fourth worst result in the country, with 19.1% of people over 15 years old, unable to read or write. Of the 312,063 inhabitants of the Bacanga river basin, 5% are illiterate.
For the calculation of the Income indicator, monthly nominal income information of all households was used for each census sector in the IBGE database for the Bacanga basin. Of the 78,154 households surveyed, 5% had no income, 87.94% had an income of up to 2 minimum wages, 5.67% had an income of 2 to 5 minimum wages, 1.09% had an income of 5 to 10 minimum wages and only 0.30% had incomes of more than 10 minimum wages.
Map of susceptibility to flooding in the Bacanga basin
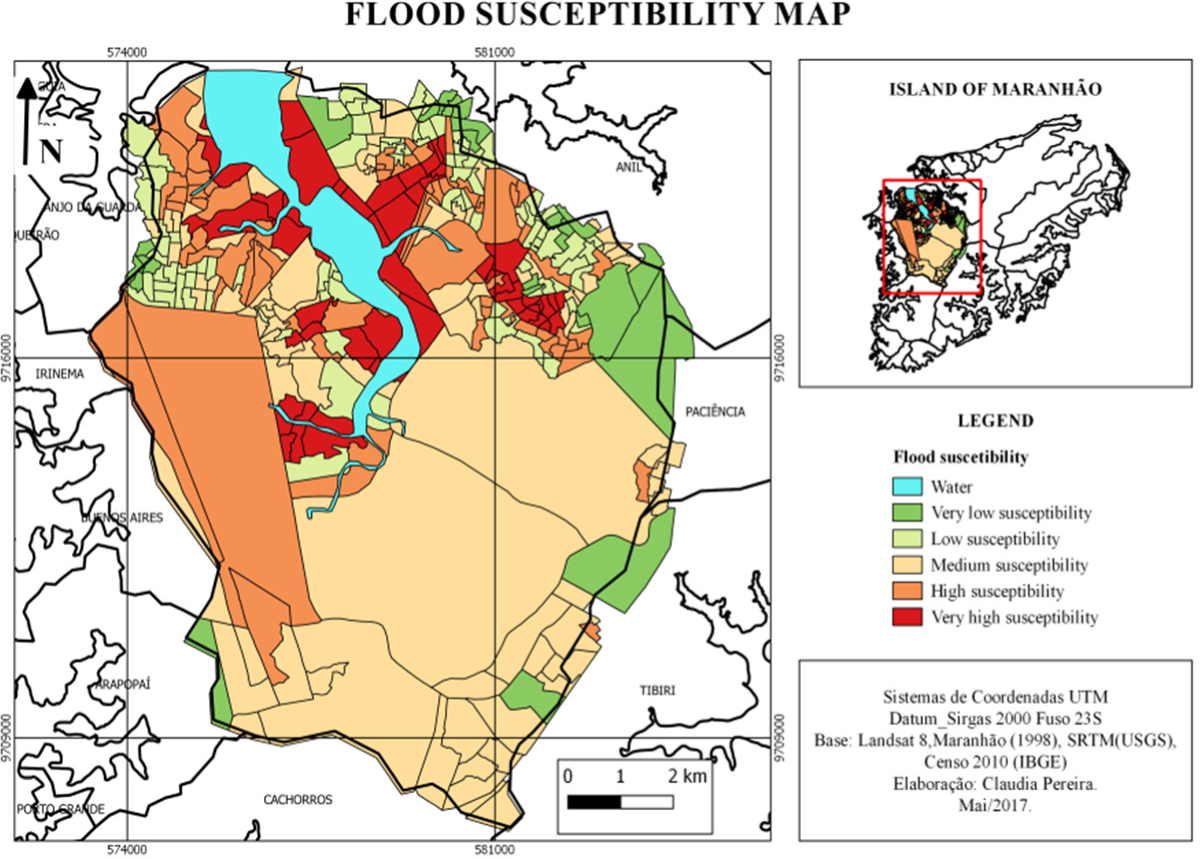
The distribution of the population of the Bacanga basin by age presents: 47.56% of the population between 20 and 50 years, 31.68% of the population between < 15 and > 65 years and 20.75% of the population between 15-20 and 50-65 years.
Figure 3 shows the specialized result of the mapped vulnerabilities. From these results, something about 27% of the territory are classified as very low vulnerable – despite the pressure exerted by informal urban occupation, the basin still has a large vegetation cover, due to the Bacanga State Park and the permanent preservation area (APA) of Maracanã and an industrial region, 17% are classified as having low vulnerability, where the basin presents some urban voids close to the tributaries of the main river, 20% of the basin is classified as moderately vulnerable, in an area of the historical center of São Luís, 21% are classified as vulnerable and about 15% as very vulnerable.
Map of social vulnerability of the Bacanga watershed
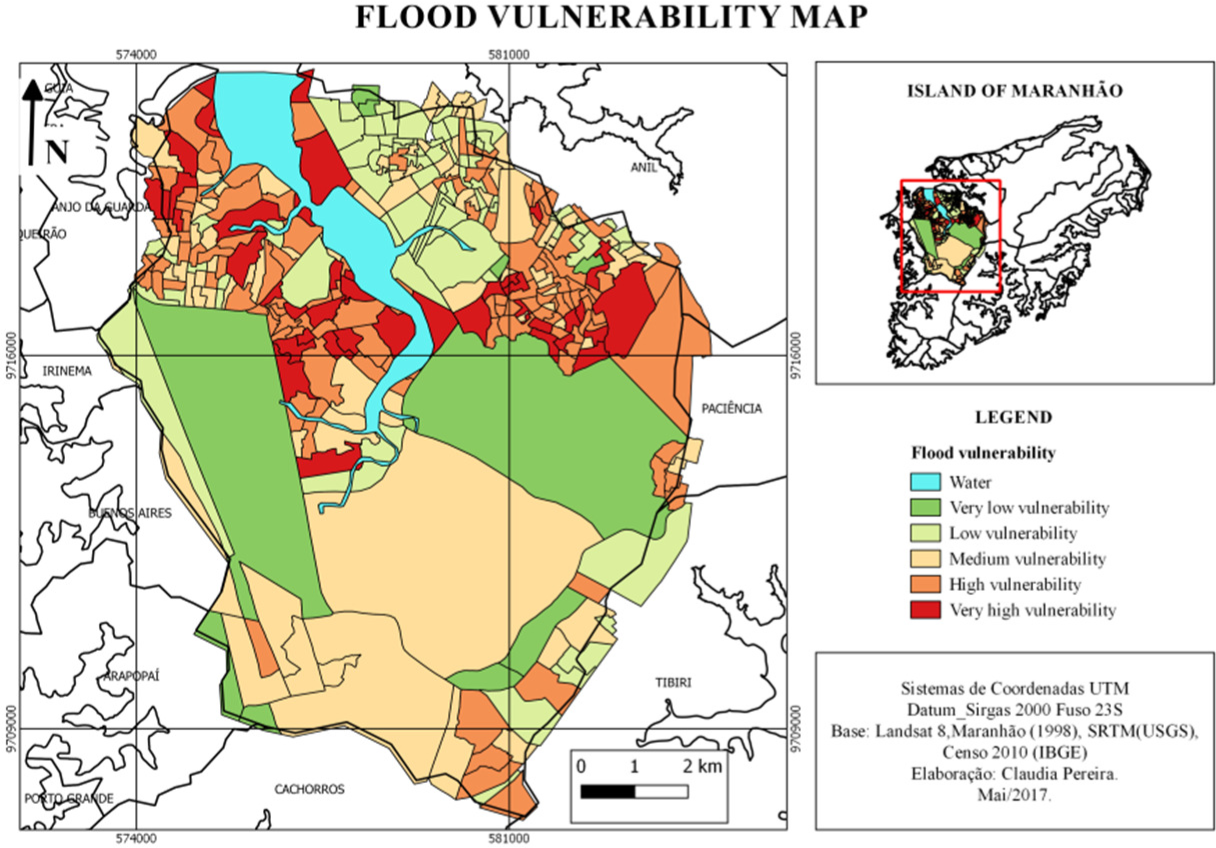
The association of the physical and social aspects of the catchment area of the Bacanga River results in a flood risk map defined by the IRI formulation.
The weights were taken as equal for both sub-indices, since it was considered that there is no risk without the susceptibility to flooding being able to reach a vulnerable area. Since both indices are associated with the same subdivisions of the domain, that is, census tracts, it is possible to easily operate them to obtain the IRI value. Figure 4 shows the results for the IRI.
It is possible to observe that the areas at greatest risk are located in the floodplain of the Bacanga River, in relatively flat and low areas. From time to time, these areas receive excess waters that overflow from the watercourses and reach the installed population there. The results show that, in a qualitative evaluation, the most critical areas are those with low slopes and with great social vulnerability, as previously expected by construction.
The flood hazard areas of the Bacanga river basin are also socially vulnerable areas. Approximately 90% of households earn less than two minimum wages. It is verified that the results obtained by the IRI express the order of magnitude of the problems associated with flooding in the study area. The weighting adopted for the selected indicators shows consistency, given the results of the simulation, although the discussion on which weights to use is always open.
Flood risk map in the Bacanga watershed
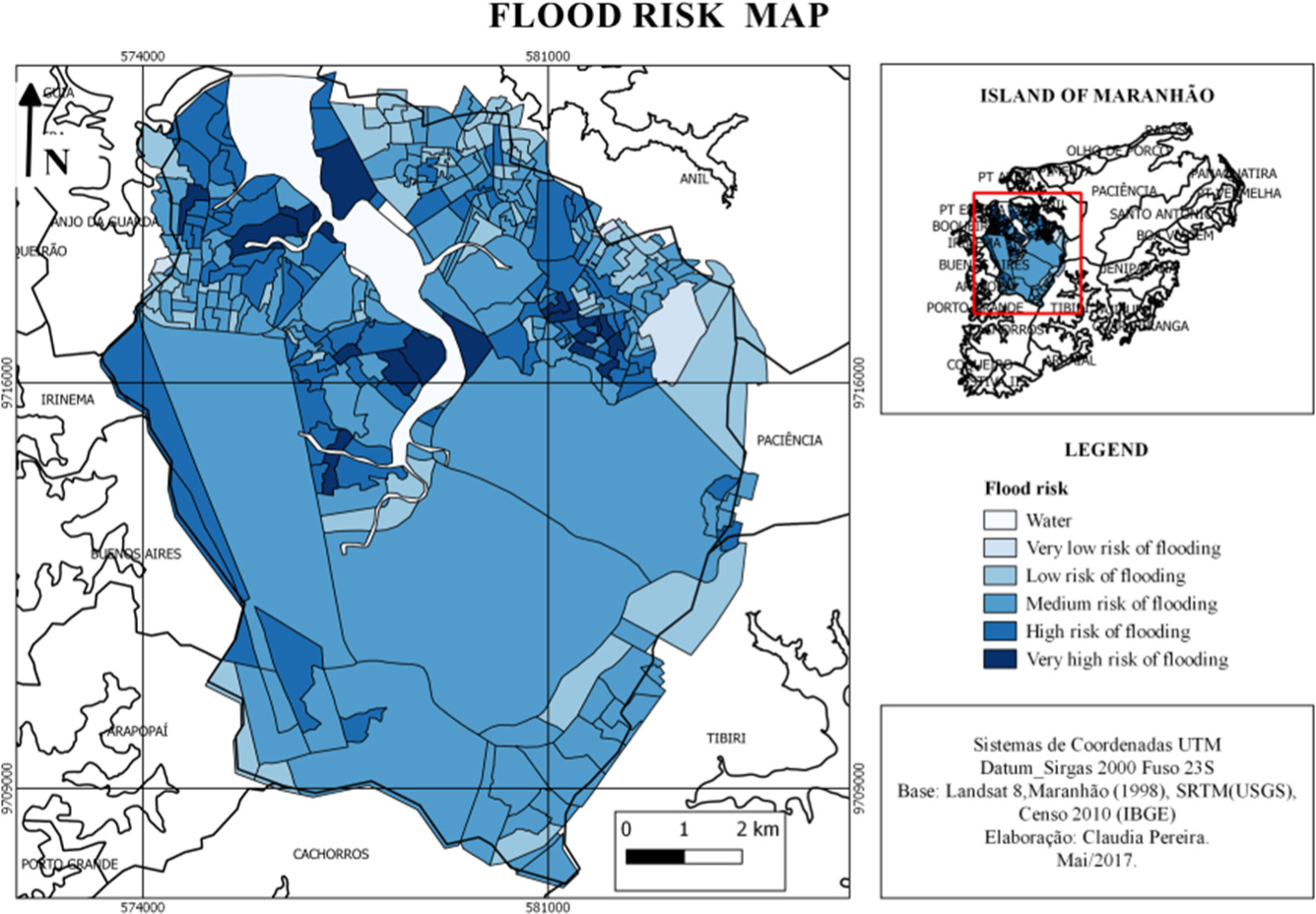
In order to validate the applicability of the proposed formulation, susceptibility to floods was compared with a hydrodynamic simulation. It should be noted that, in this model, it is possible to simply measure flooding, without quantification of effects on the socioeconomic system. Therefore, the sub-index VUL, which involves socioeconomic aspects cannot be measured by the model. Figure 5 shows the flood map obtained with MODCEL for the current urbanization conditions of the Bacanga River basin, for actual rainfall of April 11, 2009, resulting in a total precipitation of 155.6 mm. The time of concentration of the Bacanga watershed is 3.5 hours. This time was considered as equal to the duration time of the rainfall event, and the temporal distribution was defined by applying the Alternate Blocks Method, also called the Bureau of Reclamation Method [44], in order to supply this missing information, once only the total rainfall was measured.
In the IRI application, the susceptibility to flooding of the physical environment, represented by the SUS sub-index (which measures the hazard and therefore has a conceptual analogy with the flood mapped by a hydrodynamic model) can be compared with the result of Figure 5, for purposes of validation of the indirect representation that was proposed. In this way, the result of the proposed index can be considered satisfactory, since it is compatible with the modeled results, which represent observed reality. The SUS results showed good adherence to the results of the MODCEL hydrodynamic model. Note that the water level classes in which this event was mapped are presented in Table 13.
Flood of the Bacanga/Rain river (11/04/2009)
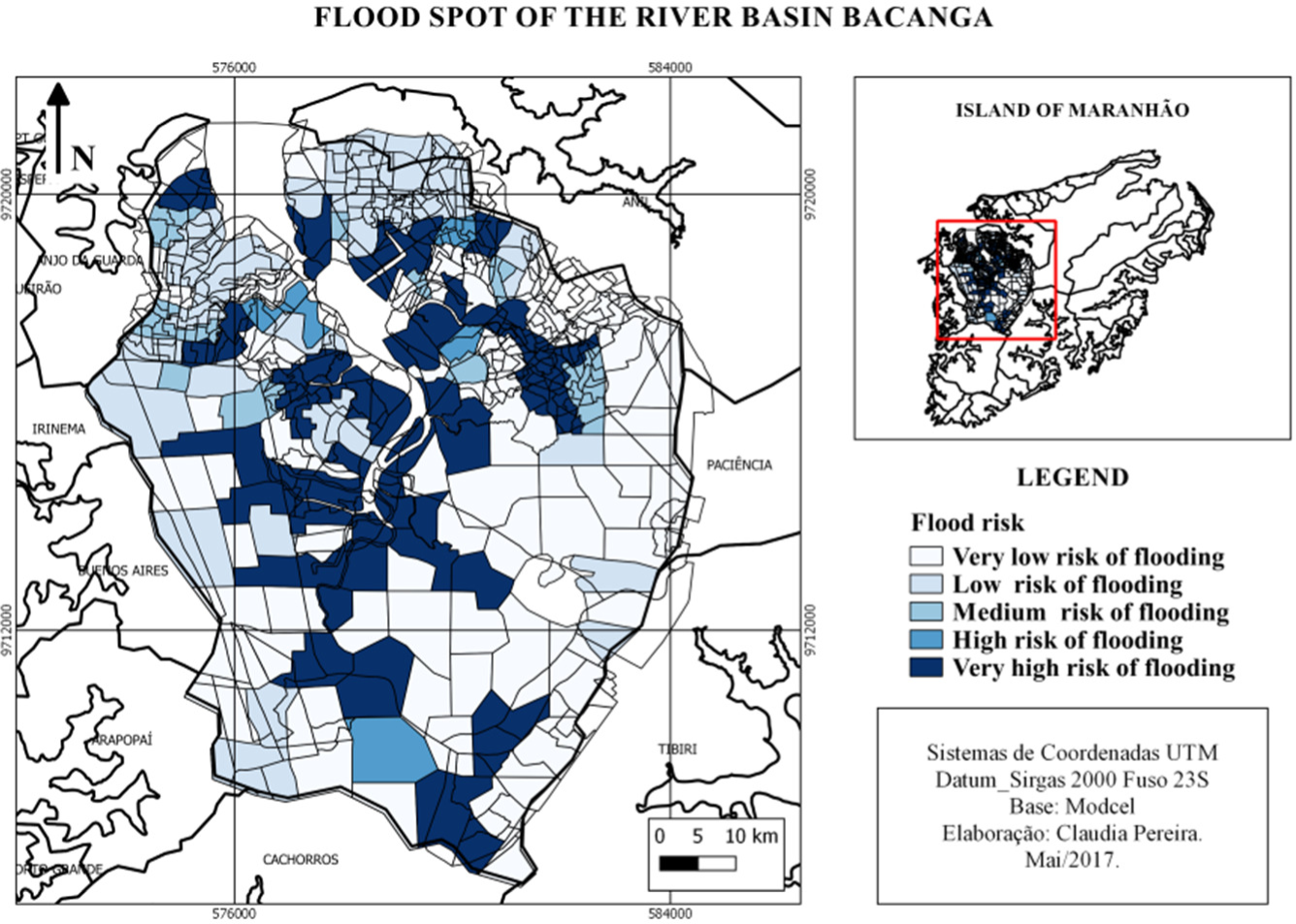
Flood level classes
Classes | Water levels |
---|---|
Very low flood levels | 0.00-0.10 |
Low flood levels | 0.10-0.20 |
Medium flood levels | 0.20-0.35 |
High flood levels | 0.35-0.50 |
Very high flood levels | More than 0.50 |
The IRI results show that the areas with the most critical risk values are also the most urbanized, with subnormal clusters and with high demographic density, in addition to being located near the watercourses. When compared to the flood map presented in the cell model, IRI result shows that the population density indicator ends up incorporating critical effects, even in areas with higher altimetry where the flood hazard is not likely to occur.
IRI appears to be a useful tool for the interpretation of the territory, with respect to its propensity to flood and to evaluate (albeit qualitatively) the effects of these possible floods on society.
The IRI establishes a multi-criteria methodology for determining the risk of flooding in a simple way, using easily available data, avoiding more sophisticated tools of mathematical modeling in the planning stage.
The study carried out in the Bacanga river basin, located in the city of São Luís do Maranhão, shows the effectiveness of the proposed and developed index. In addition, the subscript referring to the natural characteristics of the basin, whose statement pretends to simulate the physical susceptibility to floods, is corroborated by the MODCEL hydrodynamic model, used to produce flood maps for the studied area.
With regard to the indicators chosen for the composition of the index, they worked in a satisfactory and representative way. Its characteristics allowed to spatialize areas prone to flooding, so that the greater the susceptibility of the region associated with the social vulnerability of the population, the greater the risk.
The normalization scales were elaborated in an intuitive way, using indirect references, constituting a possible fragility of this study, which suggests that other alternatives can be tested. It is recommended to add a sensitivity test to the study, for weights and also for the proposed scales. These aspects, however, do not invalidate the general methodology.
Both the formulation and the composition of the index are positive points of this methodology, since each sub-index represents the main components of risk assessment. The formulation clearly works in associating hazard and vulnerability through a product that makes risk null if one of these components is null, and the indicators use simple data usually available at the municipal level.
It should also be emphasized that the use of GIS for data storage, analysis and processing proved to be very convenient and efficient, as it allowed combining, analyzing and visualizing several types of information in the same area, allowing the results to be presented clearly and adequately, even for a non-specialized audience.
By identifying areas at risk of flooding, IRI can assist in the formulation of guidelines, regulations and legal standards to land use and urban growth planning, in addition to indicating areas already occupied and fragile, that demand attention and mitigation actions from the public power.
Flood Risk Assessment on Selected Critical Infrastructure in Kota Marudu Town, Sabah, Malaysia ,MATEC Web of Conferences , Vol. 103 (4019),pp 1-9 , 2017, https://doi.org/https://doi.org/10.1051/matecconf/201710304019
, Urban Flood Disaster Management ,Procedia Eng. , Vol. 32 ,pp 25-37 , 2012, https://doi.org/https://doi.org/10.1016/j.proeng.2012.01.1233
, Assessment of Pluvial Flood Exposure and Vulnerability of Residential Areas ,Int. J. Disaster Risk Reduct. , Vol. 21 ,pp 367-375 , 2017, https://doi.org/https://doi.org/10.1016/j.ijdrr.2017.01.016
, - , Language of Risk – Project Definitions, Flood Site Report T32-04-01, 2005
- 2009 UNISDR Terminology on Disaster Risk Reduction, 20092019, https://www.unisdr.org/files/7817_UNISDRTerminologyEnglish.pdf
- , The Risks: Man as Aggressor and Environmental Victim (in Portuguese), 2007
- , , World Risk Report 2014, 2014
- , Climate Change 2007: Impacts, Adaptation, and Vulnerability: Contribution of Working Group II to the Fourth Assessment Report of the Intergovernmental Panel, 2007
Smart Adaptation to Flooding in Urban Areas ,Procedia Eng. , Vol. 118 ,pp 1096-1103 , 2015, https://doi.org/https://doi.org/10.1016/j.proeng.2015.08.449
, Lagos (Nigeria) Flooding and Influence of Urban Planning ,Proc. Inst. Civ. Eng. Urban Des. Plan. , Vol. 164 (3),pp 175-187 , 2011, https://doi.org/https://doi.org/10.1680/udap.1000014
, Learning and Action Alliances for the Integration of Flood Risk Management into Urban Planning: A New Framework from Empirical Evidence from the Netherlands ,Environmental Science & Policy , Vol. 14 (5),pp 543-554 , 2011, https://doi.org/https://doi.org/10.1016/j.envsci.2011.04.006
, A Theory on Urban Resilience to Floods – A Basis for Alternative Planning Practices ,Ecology and Society , Vol. 17 (4),pp 15 , 2012, https://doi.org/https://doi.org/10.5751/ES-05231-170448
, Challenges in Urban Flood Management: Traveling Across Spatial and Temporal Scales ,Journal Flood Risk Management , Vol. 1 (2),pp 81-88 , 2008, https://doi.org/https://doi.org/10.1111/j.1753-318X.2008.00010.x
, - , , Bachelor Thesis, 2017
Profiling Urban Vulnerabilities to Climate Change: An Indicator-Based Vulnerability Assessament for Europan Cities ,Ecological Indicators , Vol. 78 ,pp 142-155 , 2017, https://doi.org/https://doi.org/10.1016/j.ecolind.2017.02.040
, Flood Risk Perception and Adaptation Capacity: A Contribution to the Socio-Hydrology Debate ,Hydrol. Earth Syst. Sci. , Vol. 21 (6),pp 3183-3198 , 2017, https://doi.org/https://doi.org/10.5194/hess-21-3183-2017
, A Mathematical Model for Flood Loss Estimation ,Journal Hydrology , Vol. 277 (1-2),pp 24-49 , 2003, https://doi.org/https://doi.org/10.1016/S0022-1694(03)00084-2
, Volunteered Geographic Information for Disaster Management with Application to Rapid Flood Damage Estimation ,Geomatica , Vol. 64 (1),pp 89-98 , 2010, https://doi.org/https://doi.org/10.1057/fsp.2010.5
, - , Flood Risk Assessment and Coping Capacity with Flood in Central Vietnam, 2014
- , , 2006
Retrieval Effectiveness of Controlled and Uncontrolled Index Terms in 87yu6INSPEC Database ,Malaysian J. Libr. Inf. Sci. , Vol. 19 (2),pp 103-117 , 2014
, - , Performance Indicators for Water Supply Services: Manual of Best Pratice, 2007
Extending GIS-Based Visual Analysis: The Concept of Visualscapes ,Int. Journal Geographical Information Science , Vol. 17 (1),pp 25-48 , 2003, https://doi.org/https://doi.org/10.1080/713811741
, Development of 2D Map and 3D Model of GIT Campus Using GIS Technology ,Int. Res. Journal Engineering and Technology , Vol. 4 (6),pp 2597-2601 , 2017
, Detecting Flood Susceptible Areas Using GIS-based Analytic Hierarchy Process ,Proceedings of the 2nd International Conference on Future Environment and Energy , Vol. 28 ,pp 4-8 , 2012
, Simple Spatially-Distributed Models for Predicting Flood Inundation: A Review ,Geomorphology , Vol. 90 (3-4),pp 208-225 , 2007, https://doi.org/https://doi.org/10.1016/j.geomorph.2006.10.021
, Pollution of the Black Sea and its Study by Methods of Mathematical Modeling ,Journal of the Georgian Geophysical Society , Vol. 14 ,pp 53-74 , 2010
, - , Mathematical Model of Flow Cells for Urban Basins (in Portuguese), Ph.D. Thesis, 2001
- , Risk Index as a Flood Management Tool (in Portuguese), Ph.D. Thesis, 2007
Integrated Hydrodynamic and Economic Modelling of Flood Damage in The Netherlands ,Ecological Economics , Vol. 66 (1),pp 77-90 , 2008, https://doi.org/https://doi.org/10.1016/j.ecolecon.2007.12.022
, Topography-And Nightlight-Based National Flood Risk Assessment in Canada ,Hydrol. Earth Syst. Sci. , Vol. 21 (4),pp 2219-2232 , 2017, https://doi.org/https://doi.org/10.5194/hess-21-2219-2017
, - , City and Environment: The Appropriation of the Relief in the Urban Environmental Design (in Portuguese), 2012
Preventive Actions in the Context of Social Vulnerability (in Portuguese) ,Serviço Soc. em Rev. , Vol. 15 (1),pp 108-136 , 2012
, - , The Analytic Hierarchy Process, 1980
- , Contribution of Earth Observation Data to Flood Risk Mapping, 2005
- , National Soil Survey and Conservation Service (Rio de Janeiro, RJ), 1979
- , , Characteristics of the Population and Households: Results of the Universe (in Portuguese), 2011
- , National Continuous Household Sample Survey – Continuous PNAD (in Portuguese), 2015
- , , 1998
- , The Civil Defense Law: Some Considerations (in Portuguese), 2012
- , MODCEL: A Mathematical Model for Urban Flood Simulation and Integrated Flood Control Design, 2011
Urban Flood Simulation Using MODCEL – An alternative Quase-2D Conceptual Model ,Water , Vol. 9 (6),pp 445 , 2017, https://doi.org/https://doi.org/10.3390/w9060445
, - , , Ph.D. Thesis, 2011
- , Water Measurement Manual, 2001